A study on shipowners' selection preferences in response to global sulfur restrictions based on revealed preference data
-
摘要: 建立了一个基于显示性偏好数据的航运承运人减排方案决策建模框架,从实证角度研究了承运人的实际减硫方案选择机制;基于AIS数据和其他相关数据库,采用数据挖掘方法与计量经济模型,综合考虑船舶特征、承运人特征和外部市场情况等方面共11个因素,克服了已有文献关注经济因素、忽视非经济因素的局限,系统分析了限硫令下承运人的应对措施选择。研究结果表明:11个因素均有助于解释承运人的能源选择,但其影响程度各不相同;各因素的修正效应量排序依次为距离新规实行的年限(3.957)、载重吨位(2.270)、船龄(1.711)、公司规模(1.579)、每吨燃料价差(1.456)、运价指数(1.442)、环保意识指数(1.353)、航速(1.243)、航程(1.172)、排放控制区航行占比(1.127)、贸易路线固定程度(1.108);对于承运人的能源方案选择,距离新规实行的年限和载重吨位对承运人决策产生了非常重要的影响,修正效应量均大于2.0;船龄、公司规模、每吨燃料价差、运价指数和环保意识指数5个因素对于决策的影响程度适中,修正效应量为1.3~1.8;其余4个与承运人运营模式相关因素虽然对于决策有一定影响,但影响程度较小,其修正效应量均小于1.3。Abstract: A decision-making modeling framework for the shipowners' emissions reduction plan based on the revealed preference (RP) data was established, and the selection mechanism for the shipowners' practically feasible plan for reducing the sulfur emissions was empirically investigated. Based on the AIS data and the data from other related databases, 11 factors from ship particulars, shipowner characteristics, and external market conditions were comprehensively considered. The limitations of existing literatures focusing on economic factors and ignoring non-economic factors were overcome, and the shipowners' selection of countermeasures under sulfur restrictions was systematically analyzed. Analysis results show that the 11 factors considered in this study, which exhibit varying degrees of effects, contribute to the interpretation of the shipowners' energy selection. In terms of the modified effect sizes, the factors are sequentially presented: the time period away from the implementation of the global sulfur restrictions (3.957), the deadweight tonnage (2.270), the ship age (1.711), the company size (1.579), the price difference per ton of the fuel (1.456), the freight rate index (1.442), the environmental awareness index (1.353), the speed of the ship (1.243), the voyage length (1.172), the proportion of sailing distance in the emission control area (1.127), and the trading route diversity (1.108). With regards to the shipowners' selection of an energy plan, the time period away from the implementation of the global sulfur restrictions and the deadweight tonnage significantly affect their decisions, and the modified effect sizes are more than 2.0. The five factors (i.e., the ship age, the company size, the price difference per ton of the fuel, the freight rate index, and the environmental awareness index) moderately affect their decisions, and the modified effect sizes are within 1.3-1.8. The other four factors related to the shipowners' operation patterns marginally affect their decisions, and the modified effect sizes are less than 1.3. 3 tabs, 30 refs.
-
表 1 描述性统计
Table 1. Descriptive statistics
因素 均值 标准差 使用低硫燃料 安装脱硫塔 使用低硫燃料 安装脱硫塔 船龄/年 11.205 8.475 6.607 4.013 载重吨位/百万吨 0.076 0.132 0.061 0.073 航速/kn 11.307 11.917 2.317 2.625 航程/103km 2.374 2.935 1.487 1.538 ECA中航行占比/% 0.098 0.130 0.262 0.291 贸易路线固定程度 0.732 0.739 0.145 0.140 公司规模 0.416 0.694 0.493 0.461 环保意识指数 60.504 64.874 12.523 13.220 每吨燃料价差/美元 211.158 207.380 32.845 25.401 运价指数 1.327 1.179 0.395 0.397 距离新规实行的年限 1.137 0.749 0.526 0.312 表 2 模型估计结果
Table 2. Model estimation results
因素 模型1 模型2 船龄/年 -0.094 3***
(0.009 3)-0.097 7***
(0.004 0)载重吨位/百万吨 8.30***
(0.45)11.38***
(0.31)航速/kn 0.046 5**
(0.018 0)0.087 5***
(0.008 6)航程/103 km 0.067 6**
(0.028 3)0.103 5***
(0.013 9)ECA中航行占比/% 0.311**
(0.135)0.432***
(0.067)贸易路线固定程度 0.441
(0.277)0.716***
(0.133)公司规模 0.882***
(0.086)0.919***
(0.038)环保意识指数 0.025 9***
(0.003 1)0.023 1***
(0.001 4)每吨燃料价差/美元 0.017 5***
(0.001 8)0.012 9***
(0.000 7)运价指数 -0.715***
(0.095)-0.911***
(0.046)距离新规实行的年限 -2.817***
(0.124)-2.907***
(0.054)常数项 -9.047***
(0.532)-2.951***
(0.232)观测数 216 331 20 000 麦克法登可决系数 0.160 4 0.356 7 预测正确率/% 99.68 78.88 预测错误率/% 0.32 21.12 总增益/% 0.00 28.68 百分比增益/% 0.00 80.07 AUC值 0.500 0.789 表 3 模型2中各因素的效应量与修正效应量
Table 3. Effect sizes and modified effect sizes of factors in model 2
因素 标准差 效应量 修正效应量 船龄/年 5.497 0.585 1.711 载重吨位/百万吨 0.072 2.270 2.270 航速/kn 2.489 1.243 1.243 航程/103 km 1.532 1.172 1.172 ECA中航行占比/% 0.277 1.127 1.127 贸易路线固定程度 0.143 1.108 1.108 公司规模 0.497 1.579 1.579 环保意识指数 13.087 1.353 1.353 每吨燃料价差/美元 29.252 1.456 1.456 运价指数 0.402 0.694 1.442 距离新规实行的年限 0.473 0.253 3.957 -
[1] YOST T. Container-shipping industry needs to offset $10 billion[R]. New York: AlixParners, 2019. [2] LINDSTAD H, SANDAAS I, STRØMMAN A H. Assessment of cost as a function of abatement options in maritime emission control areas[J]. Transportation Research Part D: Transport and Environment, 2015, 38: 41-48. doi: 10.1016/j.trd.2015.04.018 [3] ZIS T, ANGELOUDIS P, BELL M G H, et al. Payback period for emissions abatement alternatives: role of regulation and fuel prices[J]. Journal of the Transportation Research Board, 2016, 2549(1): 37-44. doi: 10.3141/2549-05 [4] ZHU M, LI K X, LIN K C, et al. How can shipowners comply with the 2020 global sulphur limit economically?[J]. Transportation Research Part D: Transport and Environment, 2020, 79: 102234. doi: 10.1016/j.trd.2020.102234 [5] PATRICKSSON Ø, ERIKSTAD S O. A two-stage optimization approach for sulphur emission regulation compliance[J]. Maritime Policy and Management, 2017, 44(1): 94-111. doi: 10.1080/03088839.2016.1237781 [6] BALLAND O, GIRARD C, ERIKSTAD S O, et al. Optimized selection of vessel air emission controls—moving beyond cost-efficiency[J]. Maritime Policy and Management, 2015, 42(4): 362-376. doi: 10.1080/03088839.2013.872311 [7] YANG Zai-li, ZHANG Di, CAGLAYAN O, et al. Selection of techniques for reducing shipping NOx and SOx emissions[J]. Transportation Research Part D: Transport and Environment, 2012, 17(6): 478-486. doi: 10.1016/j.trd.2012.05.010 [8] SCHINAS O, STEFANAKOS C N. Selecting technologies towards compliance with MARPOL Annex VI: the perspective of operators[J]. Transportation Research Part D: Transport and Environment, 2014, 28: 28-40. doi: 10.1016/j.trd.2013.12.006 [9] REN J Z, LVTZEN M. Fuzzy multi-criteria decision-making method for technology selection for emissions reduction from shipping under uncertainties[J]. Transportation Research Part D: Transport and Environment, 2015, 40: 43-60. doi: 10.1016/j.trd.2015.07.012 [10] YANG Dong, WU Ling-xiao, WANG Shuai-an, et al. How big data enriches maritime research—a critical review of automatic identification system (AIS) data applications[J]. Transport Reviews, 2019, 39(6): 755-773. doi: 10.1080/01441647.2019.1649315 [11] HANSEN M G, JENSEN T K, LEHN-SCHIØLER T, et al. Empirical ship domain based on AIS data[J]. Journal of Navigation, 2013, 66(6): 931-940. doi: 10.1017/S0373463313000489 [12] 廖诗管, 杨冬, 白茜文, 等. 基于船舶大数据的港口装卸效率值计算方法[J]. 交通运输系统工程与信息, 2021, 21(2): 217-223. https://www.cnki.com.cn/Article/CJFDTOTAL-YSXT202102033.htmLIAO Shi-guan, YANG Dong, BAI Xi-wen, et al. Estimation method of port handling efficiency value based on ship big data[J]. Journal of Transportation Systems Engineering and Information Technology, 2021, 21(2): 217-223. (in Chinese) https://www.cnki.com.cn/Article/CJFDTOTAL-YSXT202102033.htm [13] WINTHER M, CHRISTENSEN J H, PLEJDRUP M S, et al. Emission inventories for ships in the Arctic based on satellite sampled AIS data[J]. Atmospheric Environment, 2014, 91: 1-14. doi: 10.1016/j.atmosenv.2014.03.006 [14] ADLAND R, JIA Hai-ying, STRANDENES S P. Are AIS-based trade volume estimates reliable? the case of crude oil exports[J]. Maritime Policy and Management, 2017, 44(5): 657-665. doi: 10.1080/03088839.2017.1309470 [15] JIANG Li-ping, KRONBAK J, CHRISTENSEN L P. The costs and benefits of sulphur reduction measures: sulphur scrubbers versus marine gas oil[J]. Transportation Research Part D: Transport and Environment, 2014, 28: 19-27. doi: 10.1016/j.trd.2013.12.005 [16] NORSTAD I, FAGERHOLT K, LAPORTE G. Tramp ship routing and scheduling with speed optimization[J]. Transportation Research Part C: Emerging Technologies, 2011, 19(5): 853-865. doi: 10.1016/j.trc.2010.05.001 [17] 楼狄明, 包松杰, 胡志远, 等. 基于实船油耗与排放的拖轮航速优化[J]. 交通运输工程学报, 2017, 17(1): 93-100. doi: 10.3969/j.issn.1671-1637.2017.01.011LOU Di-ming, BAO Song-jie, HU Zhi-yuan, et al. Cruise speed optimization of tugboat based on real fuel consumption and emission[J]. Journal of Traffic and Transportation Engineering, 2017, 17(1): 93-100. (in Chinese) doi: 10.3969/j.issn.1671-1637.2017.01.011 [18] GRIMMER R, MYERS M. IMO 2020, Part 2: shipowners' perspective[R]. London: IMO, 2019. [19] LE FEVRE C. A review of demand prospects for LNG as a marine transport fuel[R]. Oxford: Oxford Institute for Energy Studies, 2018. [20] KHASAWNEH R. Tankers defer retrofits to cash in on record freight rates[R]. Singapore: Reuters, 2019. [21] YANG Dong, WU Ling-xiao, WANG Shuai-an. Can we trust the AIS destination port information for bulkships?—Implications for shipping policy and practice[J]. Transportation Research Part E: Logistics and Transportation Review, 2021, 149: 102308. doi: 10.1016/j.tre.2021.102308 [22] 刘兴龙, 初秀民, 马枫, 等. AIS报文异常动态信息甄别方法[J]. 交通运输工程学报, 2016, 16(5): 142-150. doi: 10.3969/j.issn.1671-1637.2016.05.016LIU Xing-long, CHU Xiu-min, MA Feng, et al. Discriminating method of abnormal dynamic information in AIS messages[J]. Journal of Traffic and Transportation Engineering, 2016, 16(5): 142-150. (in Chinese) doi: 10.3969/j.issn.1671-1637.2016.05.016 [23] 魏照坤, 周康, 魏明, 等. 基于AIS数据的船舶运动模式识别与应用[J]. 上海海事大学学报, 2016, 37(2): 17-22, 71. https://www.cnki.com.cn/Article/CJFDTOTAL-SHHY201602005.htmWEI Zhao-kun, ZHOU Kang, WEI Ming, et al. Ship motion pattern recognition and application based on AIS data[J]. Journal of Shanghai Maritime University, 2016, 37(2): 17-22, 71. (in Chinese) https://www.cnki.com.cn/Article/CJFDTOTAL-SHHY201602005.htm [24] JIA H Y, ADLAND R, PRAKASH V, et al. Energy efficiency with the application of Virtual Arrival policy[J]. Transportation Research Part D: Transport and Environment, 2017, 54: 50-60. doi: 10.1016/j.trd.2017.04.037 [25] IMO. Prevention of air pollution from ships[R]. London: IMO, 2011. [26] WASIKOWSKI M, CHEN X W. Combating the small sample class imbalance problem using feature selection[J]. IEEE Transactions on Knowledge and Data Engineering, 2010, 22(10): 1388-1400. doi: 10.1109/TKDE.2009.187 [27] MENARDI G, TORELLI N. Training and assessing classification rules with imbalanced data[J]. Data Mining and Knowledge Discovery, 2014, 28(1): 92-122. doi: 10.1007/s10618-012-0295-5 [28] MARTINSEN C. Alternative fuels insight platform[R]. Oslo: DNV, 2019. [29] KALYANARAMAN M. Cutting time and cost on a scrubber retrofit[R]. London: Riviera Maritime Media, 2019. [30] HSU A, ZOMER A. Environmental performance index (EPI)[J]. Wiley StatsRef: Statistics Reference Online, DOI: 10.1002/9781118445112.stat03789.pub2. -
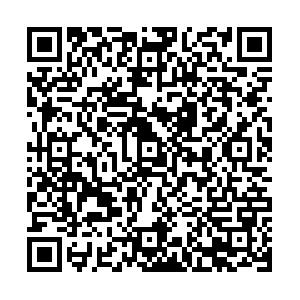
计量
- 文章访问数: 595
- HTML全文浏览量: 198
- PDF下载量: 88
- 被引次数: 0